CENSUS DATA
Problem
The agricultural sector has been disproportionately male throughout US history; however, women are becoming more active in farming and other agricultural activities. Although men still make up the majority of farmers in North Carolina, 27% of NC farmers are female and are responsible for nearly 2 million acres of agricultural land. The US Department of Agriculture has developed a Women in Agriculture Mentoring Program to help female farmers achieve their goals. We are interested in targeting counties with a lower than average percentage of female farmers to hold meetings and disseminate resources related to this USDA mentoring program. Additionally, we want to see if there is a spatial pattern of counties with below average female farmer representation to further analyze factors that may contribute to this pattern.
​
Analysis Procedures
To address this problem, I first obtained data from the US Census Bureau. I downloaded 2010 census occupation data titled “Detailed Census Occupation by Sex, and Race/Ethnicity for Residence Geography, Total Population” from the American Fact Finder website (factfinder.census.gov). The data file was titled “EEO_10_5YR_EEOALL2R_with_ann.csv”. This file included data regarding female farmers by county in North Carolina; specifically, the field titled “EST_HC1_VC9”, whose description was “Estimate; Total, race and ethnicity; Female – Percent”. I also downloaded a shapefile of North Carolina county boundaries from the TIGER/Line Shapefile website (census.gov/geo/maps-data/data/tiger-line.html). My strategy was to use ArcMap to join and map these data, as well as conduct further spatial analyses.
​
I first began by saving the census occupation data in .xlsx format and editing the table to be usable in ArcMap; for instance, removing the second descriptive column headers. Then I loaded the county boundaries data and census occupation table into ArcMap. Before joining the data, I had to add a new field to convert the “GeoID2” field from string format to double format. Then, I performed a spatial join, joining the table to the county boundaries shapefile. I then changed the symbology to display the data as graduated colors.
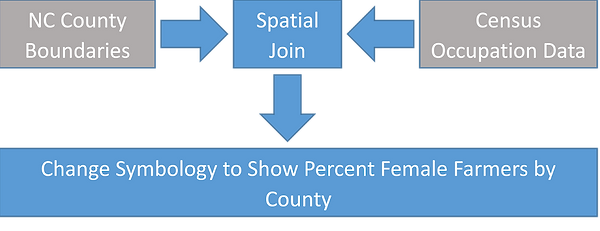
Figure 1. Diagram of methods.
Results
From an initial visual inspection, it appeared that western counties had higher percentages of female farmers, while central counties had lower percentages of female farmers. I hypothesized that there was a spacial pattern that could be statistically quantified. For the spatial analysis portion of this project, I first began by using the Average Nearest Neighbor tool to identify the overall spatial pattern. The pattern was significantly dispersed with a p-value of 0.0078, a z-score of 2.66, and a nearest neighbor ratio of 1.28. Although the pattern was dispersed, I wanted to see if there were any significant hot or cold spots as a way to identify if there were certain patterns across the state of higher or lower than average female farmers. According to this analysis, there were no significant hot spots; however, there were three counties identified as cold spots with a 90% confidence level. These cold spots included Alamance, Johnston, and Robeson counties. This analysis indicates that these counties may be best to target for the USDA mentoring program.
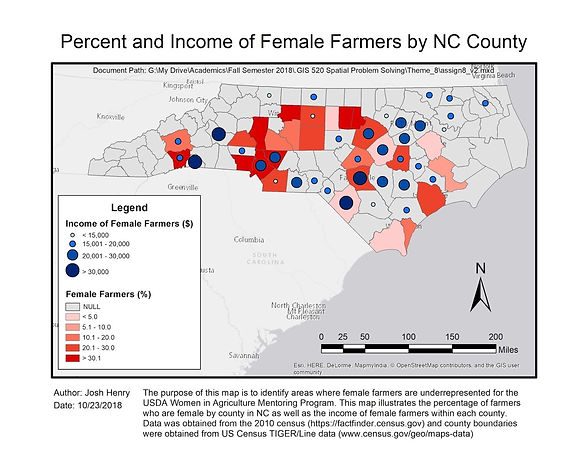
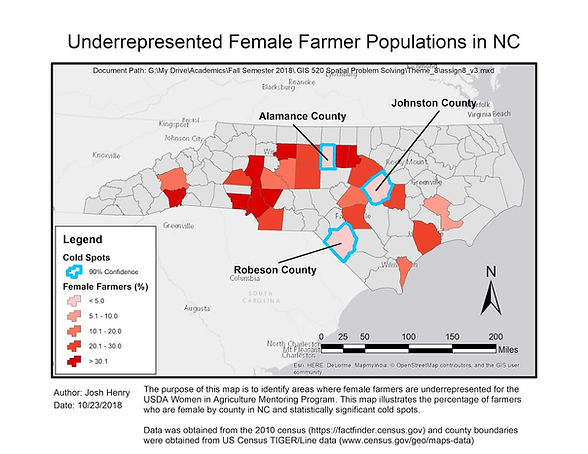.jpg)
Application & Reflection
Through this project, I learned how to search for and obtain census data and how to visualize certain fields of interest and display them in the form of a map. I also further explored the spatial pattern analysis capabilities of ArcMap. Census data provides incredible potential for GIS analysts to visualize various parameters about the US population.
​
Problem Description: Another scenario in which these procedures could be used would be determining what percentage of the working population is involved in agriculture by state.
​
Data Needed: The only data required for this analysis would include the nationwide detailed occupation census as well as a nationwide map divided by state.
​
Analysis Procedures: Using the nationwide version of the data used in this project, one could easily map this and determine which states have higher or lower than average agricultural populations. One could go even further and analyze the spatial distribution of agricultural states using the average nearest neighbor tool.